How do you collect the full potential of your data? Networking the various stakeholders is the most promising way to achieve high-quality results with fast, agile work. This is how to report recipients to become shapers with facts. Data Mesh points the way to collaboration at eye level. Haven’t you heard about the Data Mesh concept yet? I would be happy to give you a brief introduction.
You probably know the situation: It’s Wednesday, and numerous bad news has arrived in the last few days: Orders had to be canceled, the next Corona wave is building up, and the development in winter is more than unclear due to the uncertain energy supply. You will find most of the data in the data warehouse. But it is not so easy to interpret the various queries “correctly” and to incorporate them into new analyses.
To quickly prepare the right decisions here, you need high-quality data products as input and the ability to combine, organize and analyze data yourself. Depending on the questions and your previous knowledge, this will differ from your colleagues.
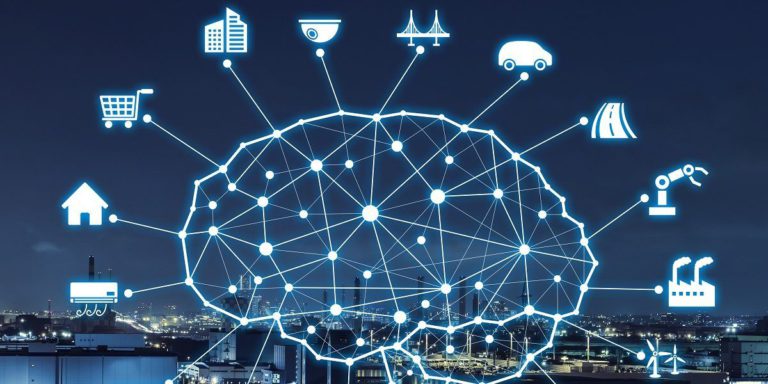
Today, data is ubiquitous and collected in many digital actions. According to Statista, the total volume of data in 2020 was 64.2 zettabytes, which is predicted to increase to 181 zettabytes per year by 2025 (hbs). In this atmosphere, one thing is clear: data is the new oil of the digital economy. And robust data analytics enables organizations to identify unique patterns from complex data sets that help them make rapid and optimal decisions in an increasingly dynamic marketplace and economy.
Fortunately, the Data Mesh architecture offers a way out of this situation. Data Mesh was first developed by Zhamak Dehghani, who was working at Thoughtworks at the time of its initial release. It leverages the principles of modern software engineering and lessons learned from building robust, Internet-based solutions to unlock the true potential of enterprise data. This architectural paradigm is now taking the industry by storm.c
Obsolete data platforms and their shortcomings
Until now, companies have typically relied on data warehouses and data lakes to store large amounts of data for analytics purposes. As a result, the analytic data plane has typically been split between these two main architectures and technologies. Data warehouses serve as repositories for structured, filtered data and then support access patterns for analytical and business intelligence reports. Data Lakes, on the other hand, hold vast pools of raw data so that Data Science can come into play. Both platforms were each under centralized responsibility. As a result, every change had to be approved through an elaborate change process, and considerable time was lost before going live.
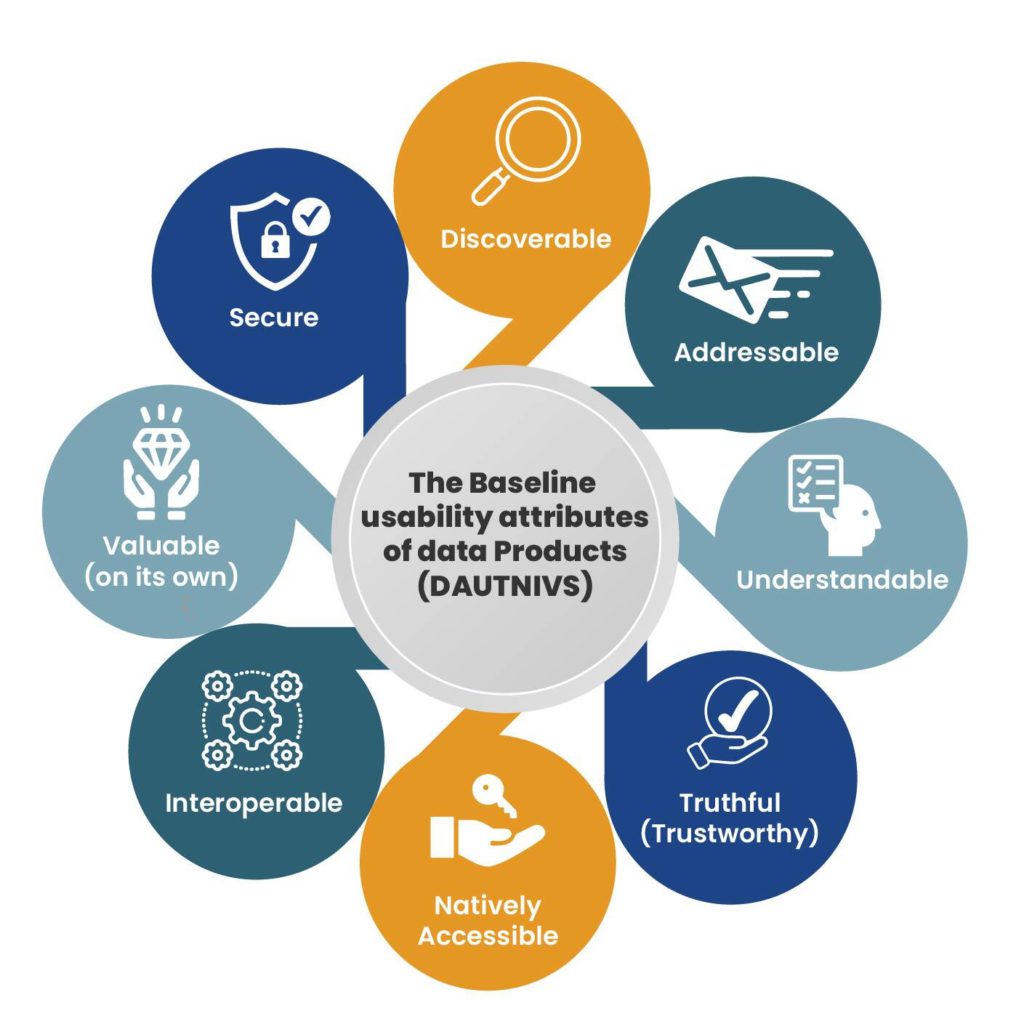
For decades, these platforms have been the dominant paradigm for data management. This type of data infrastructure generates centralized and standardized operational data that is then returned to the domain owner. This type of data architecture aims to consolidate data from across the enterprise and from external data providers and transform this raw data into cleansed, well-organized, aggregated data that can be used for reporting, analytics, feature creation and modeling.
Although the domain-oriented design has been successfully created over the years with the limited context in operational systems, data platforms have evolved from domain-oriented data ownership to centralized, domain-independent data ownership.
While this centralized model is sufficient for organizations with simpler domains and a small number of different analysis cases, organizations with extensive domains, a large number of sources, and a large number of consumers run up against the limitations of a centralized approach:
- Although organizations have historically relied on a centralized strategy for processing complex data sets from multiple sources, this method requires users to import data from edge locations into a central data lake to query for analytics, which is time-consuming and expensive.
- As global data volumes continue to grow exponentially, the query method in centralized management cannot respond sufficiently, slowing business operations. Ultimately, business agility is impacted by response time delays.
- Data transfer is often subject to various data protection guidelines. Not only do they prohibit data migration, but they also require that the data be stored in a specific location. For example, data transfer can be cumbersome if the data is stored in an EU country and a user in Japan needs to access it. Again, business agility is compromised because complying with data governance regulations and finding legal loopholes and approvals for data transfer is tedious, expensive, and time-consuming.
- Today’s enterprises have to make many complex decisions that the data architecture developed in the 1990s can no longer cope with. With data warehouses, in particular, we are seeing declining enterprise agility. These data management tools are very cost-intensive because they require a high level of management effort.
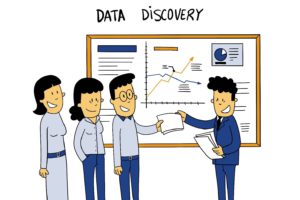
Automatisierten Berichten und Zusammenfassungen mangelt es oft an wirklichen Einblicken und Details. Die mangelnde Flexibilität der Infrastruktur bedeutet, dass es in der Regel eine erhebliche Verzögerung zwischen den Bedarf an Antworten und neuen Berichten gibt. Selbst wenn Details verfügbar sind, liegen die Daten möglicherweise nicht in einem Format vor, das eine besser lesbare Analyse im Zeitverlauf ermöglicht. Kurzum, Data Warehouses sind für neue ad hoc Fragestellungen nicht geeignet.
What distinguishes the Data Mesh architecture?
The challenges outlined call for a new way of thinking, and this is where Data Mesh comes in.
Data Mesh addresses the ubiquity of data in the enterprise by employing a domain-oriented, self-managing design. The goal is to make data readily available and interconnected within an enterprise. Although the idea may seem a bit abstract, a great analogy for Data Mesh is our nervous system: it consists of the brain and a network of interconnected independent products (organs).
Let’s look at the four principles of Data Mesh to understand how they work:
1. Domain ownership
Split data by business domain, to the business areas closest to the data – either the source of the data or its main consumers. Decompose the (analytical) data logically and based on the business domain it represents, and manage the lifecycle of the domain-oriented data independently.
3. Self-service data platform
Data is available virtually everywhere in the company in a data network. For example, let’s say you want to create a sales forecast for a particular product in a Japanese market. In this case, ideally all the data you need for a meaningful report should be available within minutes. You don’t want to wait until your requirement is prioritized, scheduled, and implemented.
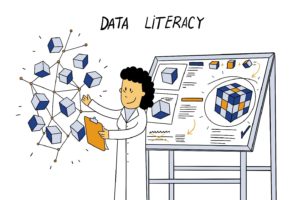
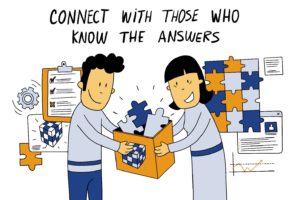
2. Data as a product
Data is considered a product owned by the team that publishes it. Data Mesh requires subject teams to take responsibility for their data. The team owns this data and must ensure the quality, coherence, and presentation of its data. It is only through the application of the data products that it becomes apparent whether the development process has been successful. Data products should not satisfy the developers, but should justify themselves through application. This principle projects a philosophy of product thinking onto analytical data.
4. Federal data governance
The main goal of this principle is to create a data ecosystem that complies with organizational rules and industry regulations while ensuring the interoperability of all data products. In the interplay of the various principles, it becomes clear that a major challenge lies in a powerful framework that ensures that the high requirements are implemented as far as possible in an automated manner. Issues such as data protection, data lineage, and uniform interfaces must be considered and tested before implementation. The decentralized responsibility and development of the various data products creates the risk of data silos or unresolvable dependencies between the individual products.
Why should companies adopt a data mesh architecture? The answer is simply that this type of data organization is best suited to modern business needs and overcomes many challenges.
- The decentralized data ownership model shortens the time to first insights and time to value by enabling business units and operational teams to quickly and easily access and analyze “non-core” data. In other words, businesses become more agile and flexible.
- The data interweaving architecture helps companies make real-time decisions by minimizing the temporal and spatial void between an event and its analytics processing. The business model becomes much more efficient and responds faster to changing trends.
- Data Mesh also overcomes the shortcomings of data warehouses and data lakes by giving data owners more autonomy and flexibility, and more data experimentation. It also reduces the burden on data teams to meet the needs of all data consumers through a single pipeline.
Feasibility – the key to success
Should this new revolutionary data organization be implemented or not? To determine whether an investment in a data mesh architecture is worthwhile, organizations need to consider the number of data sources, the size of the data teams, the number of data domains, and data governance. In general, the more extensive and complex these factors are, the more demanding your organization’s data infrastructure requirements will be, and the more likely your organization is to benefit from a data mesh approach.
In general, moving to a data mesh architecture is a sensible consideration for teams that need to handle large volumes of data sources and process them into clean data. However, if your organization’s data needs are low-complexity and sophisticated, you may not want to consider data mesh just yet. For organizations that are rapidly evolving and adapting to data modernization, it makes more sense to adopt some data mesh best practices and concepts first to facilitate migration at a later date.