In today’s digital age, the success of any business lies in leveraging its data and transforming it into actionable insights. However, this requires the implementation of a comprehensive data strategy.
Yet even as companies invest more heavily in data and analytics initiatives, they face old obstacles such as isolated and untrusted data, inefficient data management, and a lack of meaningful insights to successfully unleash the potential of data.
Table of Contents
Before making important decisions, I first do a Google search. After I have informed myself about the various aspects, I usually receive reasonable offers for personal concerns, which I can compare. In this way, we find the right offer together as a family quite quickly. In a business environment, on the other hand, it is not so easy to make the right decisions. If I have the same preferences and the same questions in private matters with thousands of other consumers, it’s not quite so easy in my own professional environment.
Here, I can’t rely on others’ data; I have to use my own data to make informed, fact-based decisions.
A good guidance framework for an appropriate data strategy has been proven to help organizations overcome these obstacles and find their way to a data-centric approach.
In the following blog, we discuss the key components of a successful data strategy.
The seven crucial elements
At a minimum, the strategy should include seven essential elements to ensure maximum efficiency and effectiveness in the use of data for decision-making:
– Alignment with business strategy ↵
– Assessment of the maturity of analytics and data ↵
– Data architecture and technology ↵
– The data analytics team ↵
– Data governance↵
– Roadmap for data strategy ↵
– Culture change and acceptance
In this article, we explain each of the components in detail and show how they can help develop a comprehensive and future-proof data strategy.
What is a data strategy?
“A data strategy is a long-term plan that defines the technology, operations, people and rules needed to manage a component of the business. Every type of business today captures large amounts of raw data. However, these companies need a well-thought-out plan for data management and analysis if they are to use this information to make informed decisions. A data strategy outlines an organization’s long-term vision for capturing, storing, sharing and using their data.” (https://aws.amazon.com/de/what-is/data-strategy/)
A data strategy is not to be confused with a business strategy. Although they are definitely closely related, the focus of a business strategy is on how a company should position itself in the marketplace, while a data strategy focuses on understanding and using the company’s proprietary data.
In addition, it’s important to note that developing a successful data strategy requires more than just collecting data and using analytics to identify trends-it’s also about identifying potential “biases” that can occur when working with large data sets. Understanding these alignments enables organizations to effectively manage their data resources to produce meaningful insights. It’s also important to consider the legal aspects of data use-internal to your organization and external to other parties who may have access to your data. In other words, make sure you comply with all applicable laws, regulations and ethical guidelines.
Finally, a comprehensive data strategy should also consider the people involved in the various elements of the overall strategy. In addition to appropriate employees or contractors with the appropriate technical skills, it is equally important to create a corporate culture that welcomes change, works collaboratively toward common goals, and values data-driven decision-making processes.
The purpose of the data strategy is to identify how the entire organization can use data to make business decisions and then create a plan that links the functions of people, processes, and technology to make the plan a reality.
To what extent is a data strategy important?
A data strategy is so important to organizations because it provides a clear understanding of their data and enables better decisions. It empowers companies to become more agile, efficient, and productive by leveraging their data assets so they can gain insights, drive innovation, and improve performance.
With increased access to reliable, high-quality data, companies can identify trends early, assess customer behavior, and gain a competitive advantage over their rivals. It also improves collaboration between departments so that everyone can access the same information quickly and easily.
A data strategy is also important for ethical reasons: it shows how a company should manage its data responsibly to protect customer privacy and comply with applicable laws and regulations. It helps ensure that the data collected is used accurately, reliably, and securely to achieve the best possible customer outcomes.
Finally, a data strategy ensures that a company is not overwhelmed by information overload, but can focus on the most important data that can be used in real time to make decisions. This can help reduce costs while improving efficiency and productivity on a large scale.
The key elements of a data strategy:
1. Alignment with corporate strategy
A data strategy is only effective if it is aligned with an organization’s business objectives. To do this, you need to be clear about how data will be used to achieve those goals and create an action plan that defines how data will be collected, processed, stored, used and secured.
Aligning data strategy with business strategy requires communication between different departments within an organization. For example, if the marketing department needs access to customer data, it needs to work closely with the IT team to ensure it can access that data in a secure manner. These two teams also need to work together when it comes to how that data should be used. This includes deciding what metrics should be tracked and reported, and what insights can be drawn from that information.
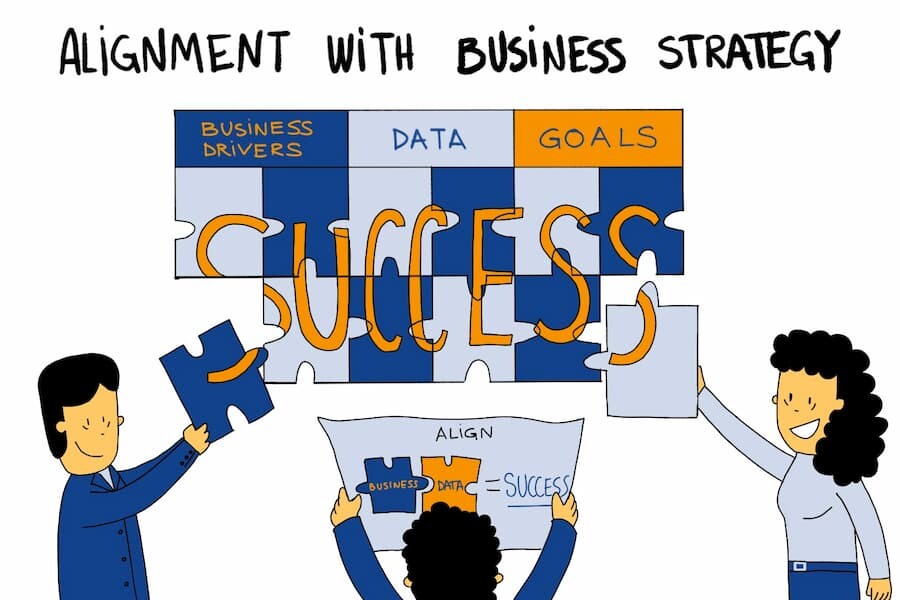
Corporate culture also plays an important role in aligning business strategy with data strategy. If employees are not encouraged or given permission,they may never realize their full potential or see opportunities for improvement and innovation. A collaborative culture where everyone feels comfortable sharing ideas and working toward common goals is beneficial to the direction of the business because it encourages the use of data to gain insights into customer behavior, product usage patterns, and other key performance indicators that can help inform decision-making at all levels of the organization.
The data strategy follows the corporate strategy
A successful data strategy should be closely intertwined with business objectives. This means that there must be clear communication between departments about which metrics are most important, how they should be used, and what should be reported on. It is beneficial to create a culture that encourages team collaboration and practical use of analytics tools so that all stakeholders can easily share ideas and quickly access the same information.
It is also important to identify any external factors that may impact the organization’s ability to achieve its goals; working with partners or customers can provide useful insights into what adjustments need to be made so that organizations and their associated data sets achieve maximum effectiveness. Establishing feedback loops can also help ensure that strategies are regularly reviewed and adjusted as needed.
When organizations have a clear idea of what type of data is needed for a particular project, they can focus their resources on gathering the most important information. This avoids wasting time and effort gathering irrelevant or unnecessary data, which could lead to inefficient decision making. It also allows companies to prioritize important tasks such as establishing privacy protocols to protect customer information from unauthorized access or misuse.
Finally, successful business and data alignment requires continuous monitoring and feedback loops; companies need to assess whether their current strategies are delivering the expected results – if not, they should adjust them accordingly until they do. Collaboration with external stakeholders such as customers or suppliers can also provide useful insights into what adjustments are needed to ensure that organizations and their associated data sets achieve maximum effectiveness.
2. Assessment of the maturity level of analyses and data
An analytic maturity assessment is important in that it gives organizations insight into the current state of their data and analytic capabilities. This allows companies to identify gaps vulnerabilities in their current systems, understand where they need to improve, and develop strategies to bring them to an appropriate maturity level.
Such an evaluation can also be used to benchmark the company within its industry or against competitors, helping executives set realistic growth goals and drive the overall data strategy for the company. By conducting regular evaluations, companies can also keep up with new technologies and consumer preferences to stay ahead of the competition.
What does it take to advance ANALYTICS in your organization? What skills and resources do you need to be successful with analytics initiatives?
For this, Tom Davenport has developed the Delta model, which he presented in the book “Analytics at Work”. The English acronym Delta stands for: Data, Enterprise, Leadership, Targets and Analysts, i.e. accessible, high-quality data, enterprise orientation, analytical leadership, strategic targets and analysts. The Greek letter “Delta” is popularly used to symbolize “change.” It is a telling image for the goal of this model.
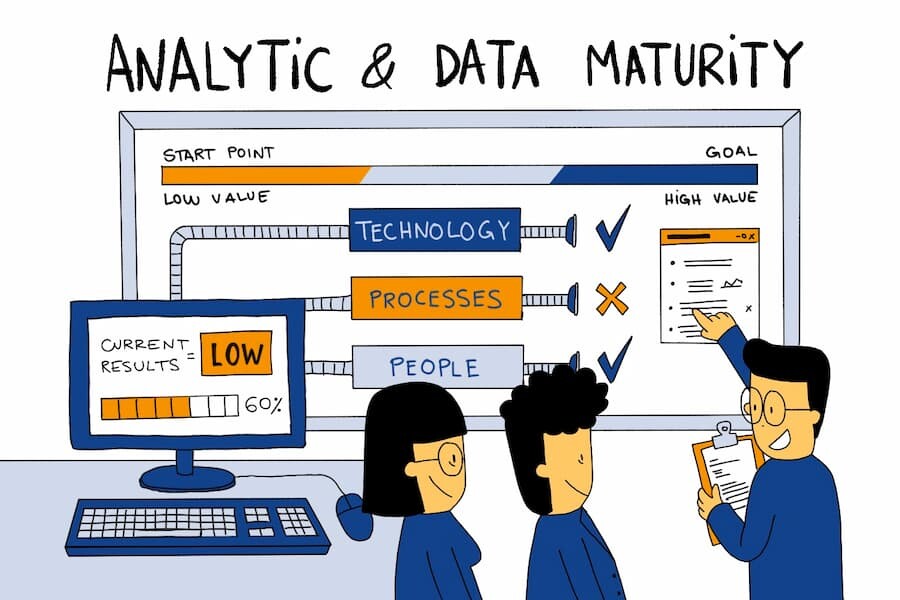
DELTA looks at the essential components from the company’s point of view. We advise to first record the current situation and to independently work out the target state. We align the elements of the data strategy with the verified target state.
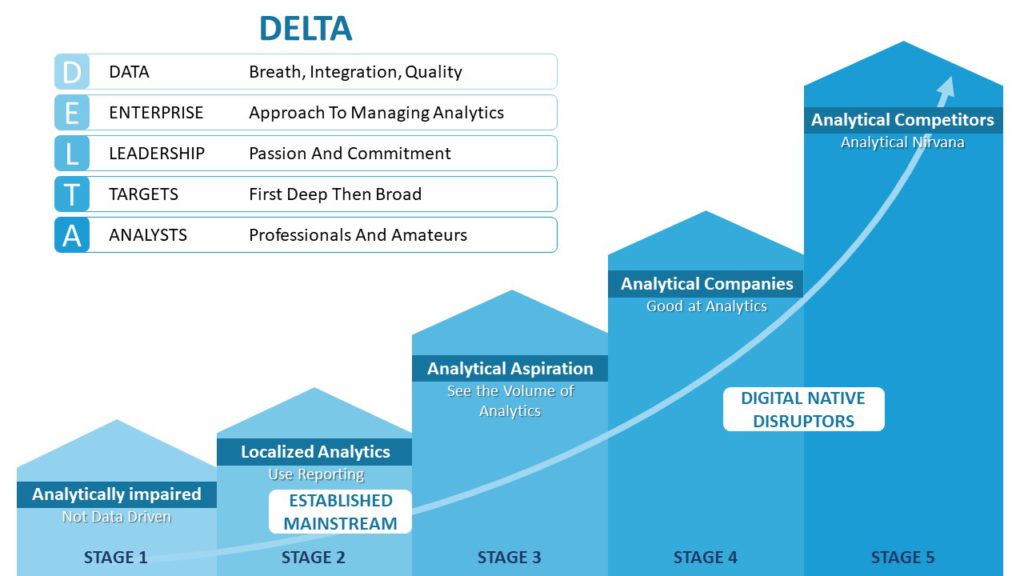
3. Data architecture and technology
Data architecture and technology are essential to any successful data strategy;without the right tools and architecture, organizations struggle to effectively capture, store, analyze and use data. This can lead to an ineffective data strategy that does not add value to the business.
Data architecture is a key component of any data strategy. It determines how your data is stored, accessed and used within the organization. For example, you can use relational databases such as SAP Data Warehouse, BW/4HANA or MS SQL Server, non-relational “NoSQL” databases such as MongoDB or Cassandra, and “Big Data” solutions, such as Hadoop or Spark. Or, you can store some of your data directly in the object store – Data Lake (for example, S3 from AWS). Each of these architectures serves a specific purpose within your overall business intelligence (BI) infrastructure. It is important to us that only those components are chosen that result in a platform from a user perspective: We follow the business analytics platform concept here.
Beyond architecture, technology also plays an important role in creating a successful data strategy. Thanks to technologies such as artificial intelligence (AI), machine learning (ML), natural language processing (NLP), blockchain-based applications, and other innovative solutions, businesses are getting deeper data insights than ever before. These technologies enable more accurate predictions about customer behavior or market trends based on historical data points; they also allow companies to automate mundane tasks that would otherwise take too much manpower or lead to errors due to manual input.
Cloud Computing
Cloud computing has additionally revolutionized the way companies store and process their data. This allows them to significantly reduce the costs associated with infrastructure and maintenance, while still delivering powerful analytics at scale. This helps companies quickly deploy new applications and integrate disparate data sets to better understand customer preferences or emerging trends in the marketplace.
In short, the right data architecture and technology is critical to a successful data strategy-it allows companies to easily access their data assets to gain insights that can be used for decision making, provides them with the ability to keep pace with emerging technologies while reducing the costs associated with infrastructure, and helps ensure that their BI platform stays in line with business goals while evolving in parallel with user needs and industry trends.
4. The data analysis team
Data analytics teams are essential for organizations to make informed decisions and develop effective data strategies. A data analytics team consists of data scientists, data engineers, and data analysts who work together to analyze and interpret large amounts of data. Data engineers design, create, and maintain data sets that can be used for data analysis. Data scientists use their expertise in mathematics, statistics, and computer science to identify recognizable patterns of behavior in the data. Data analysts then use this information to create reports and insights that can inform business decisions.
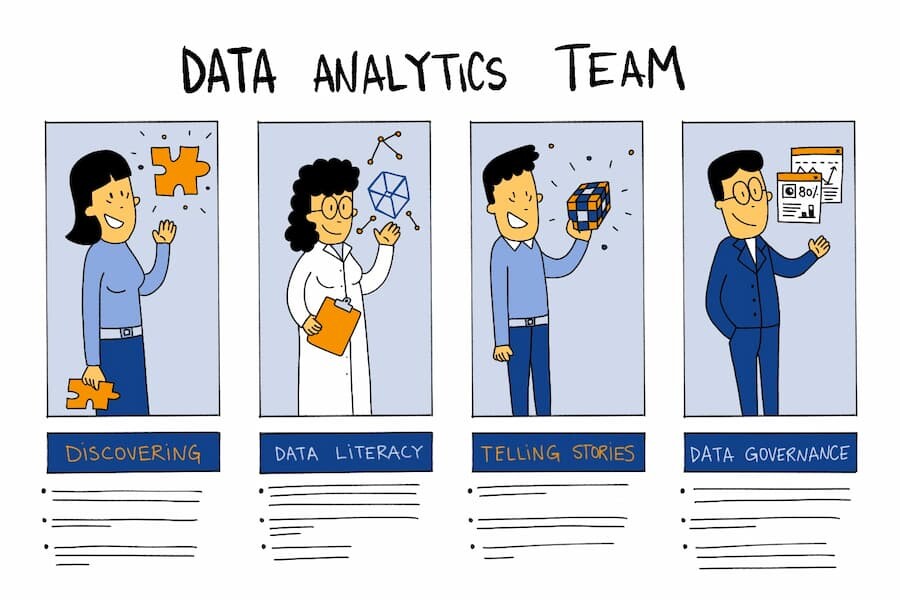
A well-structured data analytics team is the cornerstone for organizations looking to build an effective data strategy. It is important to assess the company’s current data maturity and desired end state before assembling a team of experts. This can ensure that the appropriate people with the necessary skills for the job are hired. Once the team is assembled, it is important to provide the tools and resources it needs to succeed. This includes access to reliable data sources, powerful software programs, and other technologies needed for analysis.
Data analytics teams are essential for organizations to make informed decisions and develop effective data strategies. A data analytics team consists of Data Scientists, Data Engineers, and Data Analysts who work together to analyze and interpret large amounts of data.
Data Engineers design, create, and maintain data sets that can be used for data analysis. Data Scientists use their expertise in mathematics, statistics, and computer science to identify recognizable patterns of behavior in the data. Data Analysts then use this information to create reports and insights that can inform business decisions.
A well-structured data analytics team is the cornerstone for companies looking to build an effective data strategy. It is important to assess the company’s current data maturity and desired end state before assembling a team of experts. This can ensure that the appropriate people with the necessary skills for the job are hired. Once the team is assembled, it is important to provide the tools and resources it needs to succeed. This includes access to reliable data sources, powerful software programs, and other technologies needed for analysis.
To maximize efficiency within a data analytics team, it is important that each team member clearly understands their role. Data engineers should focus on designing data sets that are easy for analysts to use while being flexible enough for future needs; analysts, on the other hand, should focus on creating reports that are useful for decision making. Furthermore, communication skills are required of all team members so that they can work effectively with each other as well as with other departments within the organization.
Developing a successful data strategy also requires thinking about how your organization will use the insights from its analytics efforts. Access to Big Data alone is not enough; you need people who can turn that information into actionable insights that can then be used by decision makers within your organization. In addition, understanding how the various departments in your organization work together and ensuring that everyone is working toward common goals set by management or stakeholders is critical to developing a comprehensive strategy.
Generally speaking, a strong data analytics team is essential to developing an effective data strategy for any organization that wants to make informed decisions based on reliable information from collected information sources. It is critical that each team member clearly understands their role so they can work together effectively while gaining useful insights into how different departments within an organization interact to achieve common goals set by management or stakeholders.
5. Data Governance
Data governance is an essential component of any successful data strategy. This involves establishing policies, procedures, and standards that support the collection, processing, and use of data within an organization. Effective data governance ensures that business data is handled securely and responsibly, while enabling its meaningful use in line with the organization’s business objectives.
Data governance can be divided into two main components: Data Management and Data Access Controls. Data management includes activities such as organizing records by category (e.g., customer demographic data), creating reusable processes for data collection and storage (e.g., backups), monitoring system performance, accurately recording changes to records (auditing), etc.
Data access control, on the other hand, is about establishing a permission framework that prohibits certain users from accessing certain data sets or performing various operations (e.g., data set deletion).
Key factor data culture
Another critical issue is creating a “data culture” in your organization where everyone is accountable for following data management and access control policies and procedures. This requires implementing training programs that educate employees about their role in complying with these rules, investing in analytics tools that facilitate enforcement of these policies, regularly reviewing the level of compliance in each department or location, etc. By ensuring that all employees understand their role in relation to data governance, you can ensure that you can protect your organization from potential risks associated with mishandling sensitive data or non-compliance with applicable regulations.
Effective security measures are also critical to a successful data strategy, as they prevent unauthorized access or data misuse. This can include technologies such as multi-factor authentication or encryption techniques. However, it should be noted that this goes beyond the use of technology – it must include physical security measures such as access control systems as well as organizational process controls such as background checks on employees before granting access rights.
Overall, proper data governance practices are essential to a successful data strategy – without them, organizations run the risk of losing valuable insights due to mishandling of their data sets or improper storage methods; they can also find themselves legally liable if they fail to meet necessary privacy or security requirements. Therefore, when planning your company’s overall strategy, establishing sound protection and usage rules for your data assets should be a top priority.
6. data strategy roadmap
Developing an effective data strategy roadmap is a critical step for any organization looking to make the most of its data assets. A working action plan includes clear goals, execution steps, and measurable milestones against which to track progress.
The first step in developing a data strategy roadmap is to define the current state and the desired state of the organization. This means assessing what data sets the company currently has access to, how they are being used, and what types of insights are being derived from them. This assessment should also consider factors such as user privacy protections, security protocols, data retention and sharing laws, etc.
Once you have identified the current state and the desired state, start planning the roadmap. Start by outlining the goals of your strategy – these should be quantitative in nature (e.g., “increase the accuracy of customer segmentation models by 20%”) so they can be easily measured over time. Work backwards from these goals to outline the steps required for implementation. Remember to plan for timelines and resources (e.g., budget or staff) that must be allocated to successfully complete each step.
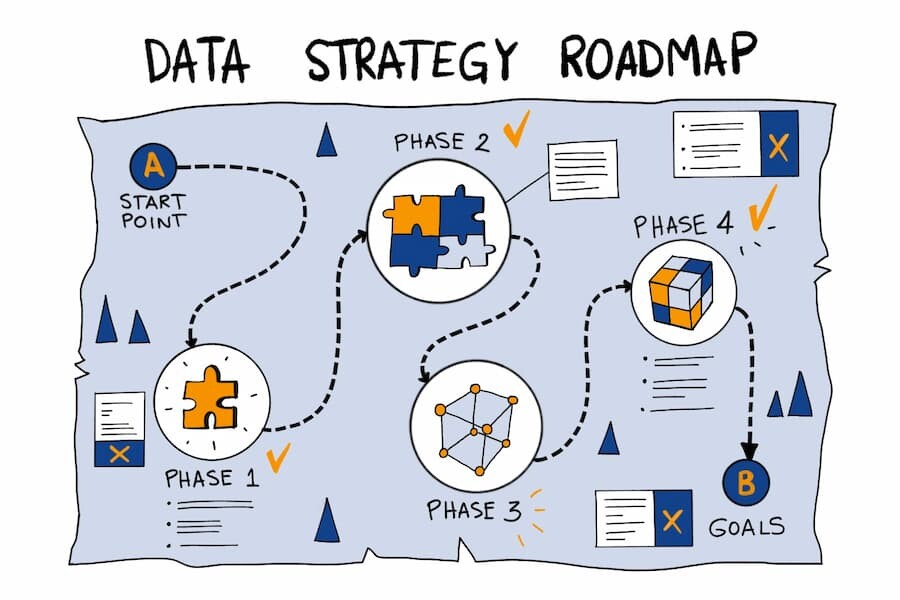
Use the project management methods for an excellent
Technical solutions (e.g., investing in new software programs or hiring additional staff) and organizational changes (e.g., establishing new rules for handling employee data) should definitely be considered when developing your plan. You should also plan for checkpoints where stakeholders can review progress at key intervals. This way, you can ensure that everyone involved is keeping an eye on the desired outcome, and give leaders the ability to adjust course as needed.
Once you’ve created a comprehensive overview of each step, it’s time to determine which actions take priority over others. When assigning priority levels, consider factors such as time constraints or interdependencies between tasks. However, don’t forget the qualitative benefits, such as improving the user experience, when deciding how quickly certain tasks should take priority over others.
Overall, developing a comprehensive roadmap requires diligence and foresight to implement a successful data strategy. Rather than focusing solely on meeting technical requirements, you should consider the impact on users, stakeholders, and other departments in the organization at all stages of development. With careful planning and thought, an effective roadmap can be created that will lead an organization to its intended goals.
7. culture change and acceptance
The data strategy has now been successfully created. Equipped with a roadmap, you are ready to proceed with data initiatives.
Last but not least, you need to address change management as your teams will face many changes and potentially new responsibilities and expectations. Without culture change, your data strategy efforts will not reach their full potential.
In God we trust, all others must bring data.
W. Edwards Deming
Fostering a data-centric culture in a company is an essential prerequisite for any successful data strategy. This starts with the example set by top management as well as executives. The quote from W. Edwards Deming illustrates an exemplary attitude. And can go as far as implementing far-reaching concepts such as Data Mesh.
In order for a company to use its data effectively, it must be ensured that all employees – from the top of the company down – are in agreement with the meaningful use of the data. This requires not only technical skills, but also that employees are properly trained, empowered and supported.
A good training concept is essential for acceptance
Der erste Schritt zur Förderung einer datengesteuerten Kultur in Ihrem Unternehmen sollte die Fortbildung und Befähigung der Mitarbeiter sein. Dazu könnte das Angebot von Kursen und Seminaren zu Themen wie der Interpretation von Berichten, die aus Datensätzen abgeleitet werden. Oder Schulungen, die Verwendung von Tools wie SAP Analytics Cloud, Tableau, Power BI oder Excel erklären und den Mehrwert aufzeigen . Ebenso wichtig ist es, dafür zu sorgen, dass alle Mitarbeiter über die erforderlichen Zugriffsrechte und Berechtigungen verfügen, um mit Datensätzen arbeiten zu können – dies verschafft die nötige Autonomie, um eigenständig Erkenntnisse zu gewinnen.
Auch das verfügbare Budget spielt eine Rolle, wenn es um die Eigenverantwortung der Mitarbeiter in Bezug auf die Arbeit mit Datensätzen geht. Während Investitionen in modernste Analysesoftware oder die Einstellung von Experten in bestimmten Bereichen kostspielig sein können, tragen selbst kleine Investitionen – wie die Bereitstellung von Mitteln für Reisekosten zur Teilnahme an Konferenzen oder Schulungsprogrammen– dazu bei, die Arbeitsmoral hochzuhalten, indem sie den Mitarbeitern zeigen, dass ihre Bemühungen vom Unternehmen geschätzt werden.
Key factor communication
And last but not least, communicating the success achieved through the use of data assets is crucial when it comes to increasing employee engagement. Successful initiatives should not go unrecognized, and making tangible results of the business strategy visible (such as revenue increases or cost reductions) not only validates the hard work of all involved, but also encourages other departments to try similar initiatives.
Overall, implementing a successful data strategy requires more than just technical know-how – it requires creating an environment where employees recognize the need to work with data sets, feel supported and empowered by their company, and receive recognition and rewards when they achieve success. With such a culture, companies ensure that they use their resources effectively and motivate their employees accordingly.
Start today
Now that you understand the importance of data strategy, take the first step and start creating your own plan. Integrate the seven key elements to maximize the value of your data assets, reduce risk and increase efficiency.
We’d love to help you develop your successful data strategy. Of course, you can continue to just put this topic off. You will have enough other things on your desk. Only then will data silos continue to emerge instead of connecting the individual solutions into a business analytics platform. Where every user, can find, understand and use the data for their question to make decisions.
Start unleashing the power of data today and create clear pathways to success.
This blog is part of a series of posts on business analytics. I recommend building a business analytics platform. The goal is to provide users with a platform for their needs, where they can find all the data and analytics tool.